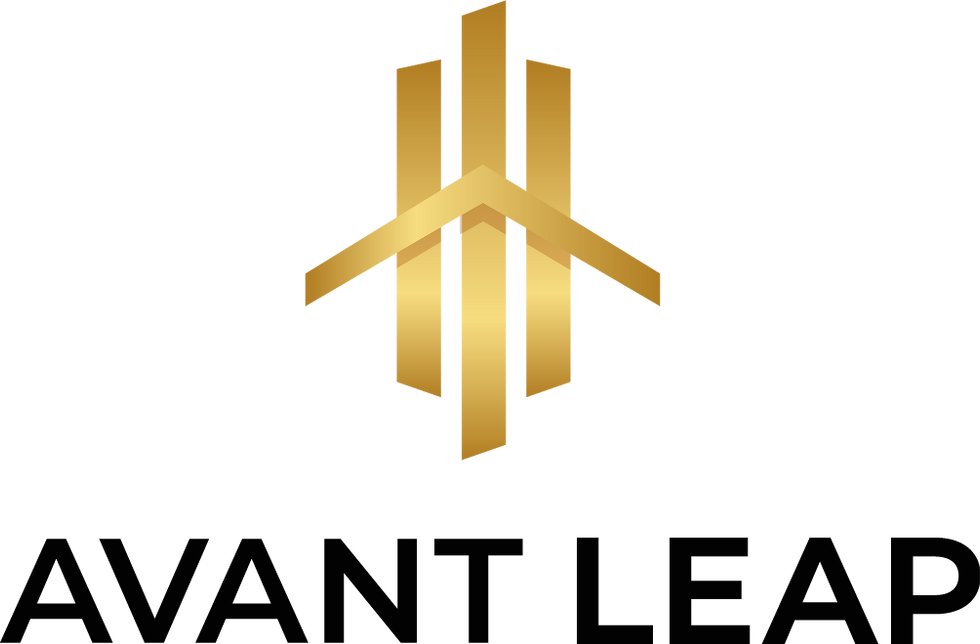
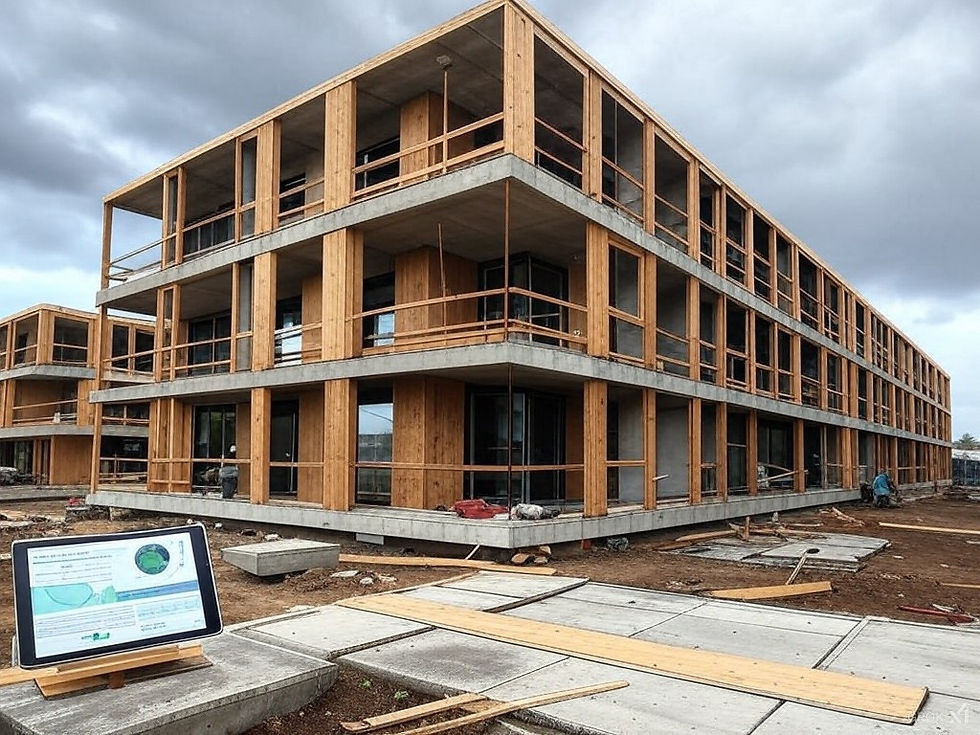
The Shift from Isolated Data to a Unified Asset Intelligence Framework
As industries expand, managing diverse assets across multiple locations becomes a balancing act between efficiency, cost, and system resilience. Traditional asset management relies on reactive maintenance, siloed monitoring, and disconnected inspections, leading to inefficiencies and unplanned downtime.
Bridging the Gap: The Role of AI in Asset Intelligence
The introduction of AI-powered Digital Twins within IoT ecosystems represents a real shift—not just as a trend but as a way to enable real-time decision-making, improve system-wide optimization, and automate engineering workflows.
This article breaks down the practical architecture behind scalable Digital Twin implementations, showing how we can integrate multi-source IoT data, AI-driven analytics, and automation frameworks to create smarter, more adaptive asset management systems.
The Digital Twin Stack: A Functional Approach to Asset Intelligence
A Digital Twin is not just a virtual model—it’s an evolving ecosystem that syncs real-time asset data with AI-powered insights. For scalability, a layered architecture ensures that data ingestion, processing, and visualization work together efficiently:
a) Data Ingestion Layer: Standardizing Multi-Source Input
To make AI-driven asset management possible, we need a unified data foundation:
IoT Sensor Integration: Collecting real-time data from industrial equipment, HVAC systems, and infrastructure sensors (temperature, pressure, vibration).
Legacy System Interoperability: Connecting SCADA, CMMS, and ERP platforms via APIs and middleware.
Data Structuring & ETL Pipelines: Standardizing data across SQL, IoT logs, JSON, and other formats to enable querying, analytics, and forecasting
b) AI-Powered Processing: From Raw Data to Intelligent Insights
Traditional AI models like anomaly detection and reinforcement learning sound great in theory—but they require massive training data, contextual accuracy, and continuous learning, which isn't always feasible for custom asset intelligence solutions. Instead, our focus is on real-time AI analysis, automation, and engineering intelligence:
Retrieval-Augmented Generation (RAG): AI systems that reference structured data from vector databases to provide intelligent, real-time insights.
AI-Driven Automation: Integrating AI agents that can read, analyze, and recommend optimizations in asset performance.
Engineering Workflow Automation: AI-enhanced MEP modeling, compliance checks, and material selection to reduce errors and improve efficiency.
c) Visualization & Decision Support: Transforming Insights into Action
Real-world asset management relies on visibility and ease of access, which is why interactive decision support tools are crucial:
Dynamic Dashboards: AI-enhanced monitoring systems displaying asset performance, predictive insights, and potential optimizations.
Stable Diffusion & Generative Models: Using AI-generated visual representations and scenario modeling for asset evaluations and performance predictions.
Material Data Analysis for Carbon Footprint: Structuring material usage data to assess environmental impact and compliance with sustainability goals.
AI-Generated Workflows: Automating work orders and maintenance schedules, reducing the need for manual intervention.
Scaling Digital Twins in Multi-Site Asset Management
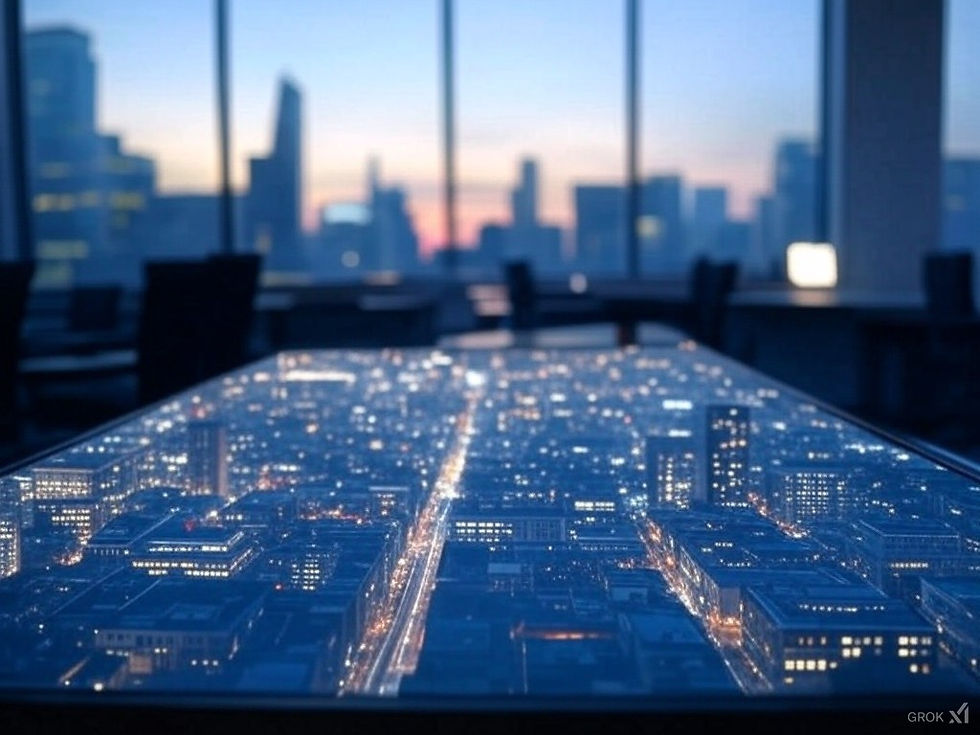
Managing assets across multiple locations presents a synchronization challenge. A truly scalable Digital Twin implementation must ensure adaptability across facilities, teams, and stakeholders. Key solutions include:
Federated AI Models: Allowing each facility to process local IoT data while contributing insights to a central AI-powered system.
Edge Computing for Real-Time Responsiveness: Local AI processing for mission-critical decisions, reducing cloud latency and response time.
Hierarchical Data Governance: Implementing role-based access controls so that teams interact with asset intelligence at the right levels (field engineers, operators, and executives)
AI in Lifecycle Optimization: Going Beyond Predictive Maintenance
A real AI-powered Digital Twin does more than just forecast failures—it actively enhances lifecycle management and adapts operations based on real-time conditions.
Energy Efficiency Optimization: AI-driven thermal modeling to reduce HVAC energy consumption in commercial buildings.
Supply Chain Adaptability: Digital Twins that adjust procurement and logistics based on real-time asset conditions and material availability.
Asset Longevity Forecasting: Predicting maintenance and replacement schedules using historical data to reduce costs and prevent premature failures.
The Strategic Shift: From Static Asset Management to AI-Powered Optimization
The transition to AI-enhanced asset intelligence isn’t just about technology—it’s about practical, scalable solutions that drive efficiency, reduce downtime, and improve decision-making.
For organizations looking to implement next-generation asset management, the key investments lie in:
Real-time IoT integration for dynamic asset tracking.
AI-powered automation to enhance engineering workflows.
Scalable Digital Twin ecosystems that synchronize across locations and teams.
Want to learn more about how AI can optimize asset intelligence in real-world scenarios? Let’s discuss how custom AI development can bring measurable impact to your workflows.
FOLLOW US:
Subscribe to our Blog to .....
Comments